Sea Ice Diagnostics for two CESM3 runs#
import xarray as xr
import numpy as np
import matplotlib as mpl
import matplotlib.pyplot as plt
import matplotlib.path as mpath
from matplotlib.gridspec import GridSpec
import cartopy.crs as ccrs
import cartopy.feature as cfeature
import cftime
import yaml
import pandas as pd
from dask.distributed import Client, LocalCluster
CESM_output_dir = "/glade/campaign/cesm/development/cross-wg/diagnostic_framework/CESM_output_for_testing"
cases = ["g.e23_a16g.GJRAv4.TL319_t232_hycom1_N75.2024.005","g.e23_a16g.GJRAv4.TL319_t232_zstar_N65.2024.004"]
lc_kwargs = {}
begyr1 = 62
endyr1 = 310
begyr2 = 62
endyr2 = 310
nyears = 60
# Parameters
CESM_output_dir = "/glade/campaign/cesm/development/cross-wg/diagnostic_framework/CESM_output_for_testing"
cases = ["b.e23_alpha16g.BLT1850.ne30_t232.075", "b.e23_alpha16g.BLT1850.ne30_t232.079"]
begyr1 = 1
endyr1 = 102
begyr2 = 1
endyr2 = 48
nyears = 25
subset_kwargs = {}
product = "/glade/u/home/dbailey/CUPiD/examples/coupled_model/computed_notebooks/quick-run/seaice.ipynb"
# Spin up cluster
cluster = LocalCluster(**lc_kwargs)
client = Client(cluster)
client
Client
Client-7e2f047e-da61-11ee-becf-ac1f6bc7cc7e
Connection method: Cluster object | Cluster type: distributed.LocalCluster |
Dashboard: http://127.0.0.1/proxy/8787/status |
Cluster Info
LocalCluster
60e7796b
Dashboard: http://127.0.0.1/proxy/8787/status | Workers: 9 |
Total threads: 72 | Total memory: 376.54 GiB |
Status: running | Using processes: True |
Scheduler Info
Scheduler
Scheduler-7430b765-bc61-4e3f-9118-ba8a35561646
Comm: tcp://127.0.0.1:37195 | Workers: 9 |
Dashboard: http://127.0.0.1/proxy/8787/status | Total threads: 72 |
Started: Just now | Total memory: 376.54 GiB |
Workers
Worker: 0
Comm: tcp://127.0.0.1:37867 | Total threads: 8 |
Dashboard: http://127.0.0.1/proxy/44105/status | Memory: 41.84 GiB |
Nanny: tcp://127.0.0.1:44317 | |
Local directory: /glade/derecho/scratch/dbailey/tmp/dask-scratch-space/worker-tujn6yhv |
Worker: 1
Comm: tcp://127.0.0.1:45597 | Total threads: 8 |
Dashboard: http://127.0.0.1/proxy/40057/status | Memory: 41.84 GiB |
Nanny: tcp://127.0.0.1:42665 | |
Local directory: /glade/derecho/scratch/dbailey/tmp/dask-scratch-space/worker-d9i9ix_z |
Worker: 2
Comm: tcp://127.0.0.1:43395 | Total threads: 8 |
Dashboard: http://127.0.0.1/proxy/40731/status | Memory: 41.84 GiB |
Nanny: tcp://127.0.0.1:45929 | |
Local directory: /glade/derecho/scratch/dbailey/tmp/dask-scratch-space/worker-08tfvrs_ |
Worker: 3
Comm: tcp://127.0.0.1:42961 | Total threads: 8 |
Dashboard: http://127.0.0.1/proxy/41809/status | Memory: 41.84 GiB |
Nanny: tcp://127.0.0.1:42433 | |
Local directory: /glade/derecho/scratch/dbailey/tmp/dask-scratch-space/worker-mfddcxss |
Worker: 4
Comm: tcp://127.0.0.1:34831 | Total threads: 8 |
Dashboard: http://127.0.0.1/proxy/43967/status | Memory: 41.84 GiB |
Nanny: tcp://127.0.0.1:44483 | |
Local directory: /glade/derecho/scratch/dbailey/tmp/dask-scratch-space/worker-2jv1hi5r |
Worker: 5
Comm: tcp://127.0.0.1:40191 | Total threads: 8 |
Dashboard: http://127.0.0.1/proxy/35583/status | Memory: 41.84 GiB |
Nanny: tcp://127.0.0.1:39433 | |
Local directory: /glade/derecho/scratch/dbailey/tmp/dask-scratch-space/worker-j5d_n5q2 |
Worker: 6
Comm: tcp://127.0.0.1:34229 | Total threads: 8 |
Dashboard: http://127.0.0.1/proxy/44545/status | Memory: 41.84 GiB |
Nanny: tcp://127.0.0.1:43243 | |
Local directory: /glade/derecho/scratch/dbailey/tmp/dask-scratch-space/worker-x8ute4ag |
Worker: 7
Comm: tcp://127.0.0.1:46063 | Total threads: 8 |
Dashboard: http://127.0.0.1/proxy/43397/status | Memory: 41.84 GiB |
Nanny: tcp://127.0.0.1:39255 | |
Local directory: /glade/derecho/scratch/dbailey/tmp/dask-scratch-space/worker-u_4un_7y |
Worker: 8
Comm: tcp://127.0.0.1:33783 | Total threads: 8 |
Dashboard: http://127.0.0.1/proxy/37263/status | Memory: 41.84 GiB |
Nanny: tcp://127.0.0.1:45093 | |
Local directory: /glade/derecho/scratch/dbailey/tmp/dask-scratch-space/worker-kziz4yzh |
# Read in two cases. The ADF timeseries are needed here.
case1 = cases[0]
case2 = cases[1]
cbegyr1 = f"{begyr1:04d}"
cendyr1 = f"{endyr1:04d}"
cbegyr2 = f"{begyr2:04d}"
cendyr2 = f"{endyr2:04d}"
ds1 = xr.open_mfdataset(CESM_output_dir+"/"+case1+"/ts/"+case1+".cice.h."+"*."+cbegyr1+"01-"+cendyr1+"12.nc")
ds2 = xr.open_mfdataset(CESM_output_dir+"/"+case2+"/ts/"+case2+".cice.h."+"*."+cbegyr2+"01-"+cendyr2+"12.nc")
TLAT = ds1['TLAT']
TLON = ds1['TLON']
tarea = ds1['tarea']
# Make a DataArray with the number of days in each month, size = len(time)
month_length = ds1.time.dt.days_in_month
weights_monthly = month_length.groupby("time.year") / month_length.groupby("time.year").sum()
#seasons = xr.full_like(months, fill_value="none", dtype="U4")
#seasons.name = "season"
#seasons[months.isin([1, 2, 3])] = "JFM"
#seasons[months.isin([4, 5, 6])] = "AMJ"
#seasons[months.isin([7, 8, 9])] = "JAS"
#seasons[months.isin([10, 11, 12])] = "OND"
#weights_season = month_length.groupby(seasons) / month_length.groupby(seasons).sum()
ds1_ann = (ds1 * weights_monthly).resample(time="YS").sum(dim="time")
ds2_ann = (ds2 * weights_monthly).resample(time="YS").sum(dim="time")
#ds1_seas = (ds1 * weights_season).resample(time="QS-JAN").sum(dim="time")
#ds2_seas = (ds2 * weights_season).resample(time="QS-JAN").sum(dim="time")
with open('cice_masks.yml', 'r') as file:
cice_masks = yaml.safe_load(file)
with open('cice_vars.yml', 'r') as file:
cice_vars = yaml.safe_load(file)
print(ds1['aice'])
<xarray.DataArray 'aice' (time: 1224, nj: 480, ni: 540)>
dask.array<open_dataset-aice, shape=(1224, 480, 540), dtype=float32, chunksize=(2, 480, 540), chunktype=numpy.ndarray>
Coordinates:
* time (time) object 0001-01-16 12:00:00 ... 0102-12-16 12:00:00
TLON (nj, ni) float32 dask.array<chunksize=(480, 540), meta=np.ndarray>
TLAT (nj, ni) float32 dask.array<chunksize=(480, 540), meta=np.ndarray>
ULON (nj, ni) float32 dask.array<chunksize=(480, 540), meta=np.ndarray>
ULAT (nj, ni) float32 dask.array<chunksize=(480, 540), meta=np.ndarray>
Dimensions without coordinates: nj, ni
Attributes:
units: 1
long_name: ice area (aggregate)
cell_measures: area: tarea
cell_methods: time: mean
time_rep: averaged
def plot_diff(field1, field2, levels, case1, case2, title, proj):
# make circular boundary for polar stereographic circular plots
theta = np.linspace(0, 2*np.pi, 100)
center, radius = [0.5, 0.5], 0.5
verts = np.vstack([np.sin(theta), np.cos(theta)]).T
circle = mpath.Path(verts * radius + center)
if (np.size(levels) > 2):
cmap = mpl.colormaps['tab20']
norm = mpl.colors.BoundaryNorm(levels, ncolors=cmap.N)
# set up the figure with a North Polar Stereographic projection
fig = plt.figure(tight_layout=True)
gs = GridSpec(2, 4)
if (proj == "N"):
ax = fig.add_subplot(gs[0,:2], projection=ccrs.NorthPolarStereo())
# sets the latitude / longitude boundaries of the plot
ax.set_extent([0.005, 360, 90, 45], crs=ccrs.PlateCarree())
if (proj == "S"):
ax = fig.add_subplot(gs[0,:2], projection=ccrs.SouthPolarStereo())
# sets the latitude / longitude boundaries of the plot
ax.set_extent([0.005, 360, -90, -45], crs=ccrs.PlateCarree())
ax.set_boundary(circle, transform=ax.transAxes)
ax.add_feature(cfeature.LAND,zorder=100,edgecolor='k')
field_diff = field2.values-field1.values
field_std = field_diff.std()
this=ax.pcolormesh(TLON,
TLAT,
field1,
norm = norm,
cmap="tab20",
transform=ccrs.PlateCarree())
plt.colorbar(this,orientation='vertical',fraction=0.04,pad=0.01)
plt.title(case1,fontsize=10)
if (proj == "N"):
ax = fig.add_subplot(gs[0,2:], projection=ccrs.NorthPolarStereo())
# sets the latitude / longitude boundaries of the plot
ax.set_extent([0.005, 360, 90, 45], crs=ccrs.PlateCarree())
if (proj == "S"):
ax = fig.add_subplot(gs[0,2:], projection=ccrs.SouthPolarStereo())
# sets the latitude / longitude boundaries of the plot
ax.set_extent([0.005, 360, -90, -45], crs=ccrs.PlateCarree())
ax.set_boundary(circle, transform=ax.transAxes)
ax.add_feature(cfeature.LAND,zorder=100,edgecolor='k')
this=ax.pcolormesh(TLON,
TLAT,
field2,
norm=norm,
cmap="tab20",
transform=ccrs.PlateCarree())
plt.colorbar(this,orientation='vertical',fraction=0.04,pad=0.01)
plt.title(case2,fontsize=10)
if (proj == "N"):
ax = fig.add_subplot(gs[1,1:3], projection=ccrs.NorthPolarStereo())
# sets the latitude / longitude boundaries of the plot
ax.set_extent([0.005, 360, 90, 45], crs=ccrs.PlateCarree())
if (proj == "S"):
ax = fig.add_subplot(gs[1,1:3], projection=ccrs.SouthPolarStereo())
# sets the latitude / longitude boundaries of the plot
ax.set_extent([0.005, 360, -90, -45], crs=ccrs.PlateCarree())
ax.set_boundary(circle, transform=ax.transAxes)
ax.add_feature(cfeature.LAND,zorder=100,edgecolor='k')
this=ax.pcolormesh(TLON,
TLAT,
field_diff,
cmap="seismic",vmax=field_std*2.0,vmin=-field_std*2.0,
transform=ccrs.PlateCarree())
plt.colorbar(this,orientation='vertical',fraction=0.04,pad=0.01)
plt.title(case2+"-"+case1,fontsize=10)
plt.suptitle(title)
def vect_diff(uvel1,vvel1,uvel2,vvel2,angle,proj):
uvel_rot1 = uvel1*np.cos(angle)-vvel1*np.sin(angle)
vvel_rot1 = uvel1*np.sin(angle)+vvel1*np.cos(angle)
uvel_rot2 = uvel2*np.cos(angle)-vvel2*np.sin(angle)
vvel_rot2 = uvel2*np.sin(angle)+vvel2*np.cos(angle)
speed1 = np.sqrt(uvel1*uvel1+vvel1*vvel1)
speed2 = np.sqrt(uvel2*uvel2+vvel2*vvel2)
uvel_diff = uvel_rot2-uvel_rot1
vvel_diff = vvel_rot2-vvel_rot1
speed_diff = speed2-speed1
# make circular boundary for polar stereographic circular plots
theta = np.linspace(0, 2*np.pi, 100)
center, radius = [0.5, 0.5], 0.5
verts = np.vstack([np.sin(theta), np.cos(theta)]).T
circle = mpath.Path(verts * radius + center)
# set up the figure with a North Polar Stereographic projection
fig = plt.figure(tight_layout=True)
gs = GridSpec(2, 4)
if (proj == "N"):
ax = fig.add_subplot(gs[0,:2], projection=ccrs.NorthPolarStereo())
# sets the latitude / longitude boundaries of the plot
ax.set_extent([0.005, 360, 90, 45], crs=ccrs.PlateCarree())
if (proj == "S"):
ax = fig.add_subplot(gs[0,:2], projection=ccrs.SouthPolarStereo())
# sets the latitude / longitude boundaries of the plot
ax.set_extent([0.005, 360, -90, -45], crs=ccrs.PlateCarree())
ax.set_boundary(circle, transform=ax.transAxes)
ax.add_feature(cfeature.LAND,zorder=100,edgecolor='k')
this=ax.pcolormesh(TLON,
TLAT,
speed1,
vmin = 0.,
vmax = 0.5,
cmap="tab20",
transform=ccrs.PlateCarree())
plt.colorbar(this,orientation='vertical',fraction=0.04,pad=0.01)
plt.title(case1,fontsize=10)
intv = 5
## add vectors
Q = ax.quiver(TLON[::intv,::intv].values,TLAT[::intv,::intv].values,
uvel_rot1[::intv,::intv].values,vvel_rot1[::intv,::intv].values,
color = 'black', scale=1.,
transform=ccrs.PlateCarree())
units = "cm/s"
qk = ax.quiverkey(Q,0.85,0.025,0.10,r'10 '+units,labelpos='S', coordinates='axes',color='black',zorder=2)
if (proj == "N"):
ax = fig.add_subplot(gs[0,2:], projection=ccrs.NorthPolarStereo())
# sets the latitude / longitude boundaries of the plot
ax.set_extent([0.005, 360, 90, 45], crs=ccrs.PlateCarree())
if (proj == "S"):
ax = fig.add_subplot(gs[0,2:], projection=ccrs.SouthPolarStereo())
# sets the latitude / longitude boundaries of the plot
ax.set_extent([0.005, 360, -90, -45], crs=ccrs.PlateCarree())
ax.set_boundary(circle, transform=ax.transAxes)
ax.add_feature(cfeature.LAND,zorder=100,edgecolor='k')
this=ax.pcolormesh(TLON,
TLAT,
speed2,
vmin = 0.,
vmax = 0.5,
cmap="tab20",
transform=ccrs.PlateCarree())
plt.colorbar(this,orientation='vertical',fraction=0.04,pad=0.01)
plt.title(case1,fontsize=10)
intv = 5
## add vectors
Q = ax.quiver(TLON[::intv,::intv].values,TLAT[::intv,::intv].values,
uvel_rot2[::intv,::intv].values,vvel_rot2[::intv,::intv].values,
color = 'black', scale=1.,
transform=ccrs.PlateCarree())
units = "cm/s"
qk = ax.quiverkey(Q,0.85,0.025,0.10,r'10 '+units,labelpos='S', coordinates='axes',color='black',zorder=2)
if (proj == "N"):
ax = fig.add_subplot(gs[1,1:3], projection=ccrs.NorthPolarStereo())
# sets the latitude / longitude boundaries of the plot
ax.set_extent([0.005, 360, 90, 45], crs=ccrs.PlateCarree())
if (proj == "S"):
ax = fig.add_subplot(gs[1,1:3], projection=ccrs.SouthPolarStereo())
# sets the latitude / longitude boundaries of the plot
ax.set_extent([0.005, 360, -90, -45], crs=ccrs.PlateCarree())
ax.set_boundary(circle, transform=ax.transAxes)
ax.add_feature(cfeature.LAND,zorder=100,edgecolor='k')
this=ax.pcolormesh(TLON,
TLAT,
speed_diff,
vmin = -0.2,
vmax = 0.2,
cmap="seismic",
transform=ccrs.PlateCarree())
plt.colorbar(this,orientation='vertical',fraction=0.04,pad=0.01)
plt.title(case2+"-"+case1,fontsize=10)
intv = 5
## add vectors
Q = ax.quiver(TLON[::intv,::intv].values,TLAT[::intv,::intv].values,
uvel_diff[::intv,::intv].values,vvel_diff[::intv,::intv].values,
color = 'black', scale=1.,
transform=ccrs.PlateCarree())
units = "cm/s"
qk = ax.quiverkey(Q,0.85,0.025,0.10,r'10 '+units,labelpos='S', coordinates='axes',color='black',zorder=2)
plt.suptitle("Velocity m/s")
with open('cice_vars.yml', 'r') as file:
cice_vars = yaml.safe_load(file)
for var in cice_vars:
print(var,cice_vars[var])
vmin=cice_vars[var][0]['levels'][0]
vmax=cice_vars[var][0]['levels'][-1]
levels = np.array(cice_vars[var][0]['levels'])
print(levels)
title=cice_vars[var][1]['title']
field1 = ds1_ann[var].isel(time=slice(-nyears,None)).mean("time").squeeze()
field2 = ds2_ann[var].isel(time=slice(-nyears,None)).mean("time").squeeze()
plot_diff(field1,field2,levels,case1,case2,title,"N")
aice [{'levels': [0.05, 0.1, 0.15, 0.2, 0.3, 0.4, 0.5, 0.6, 0.7, 0.8, 0.85, 0.9, 0.95, 0.99]}, {'title': 'Sea Ice Concentration'}]
[0.05 0.1 0.15 0.2 0.3 0.4 0.5 0.6 0.7 0.8 0.85 0.9 0.95 0.99]
hi [{'levels': [0.05, 0.1, 0.25, 0.5, 0.75, 1.0, 1.5, 2.0, 2.5, 3.0, 3.5, 4.0, 4.5, 5.0]}, {'title': 'Sea Ice Thickness (m)'}]
[0.05 0.1 0.25 0.5 0.75 1. 1.5 2. 2.5 3. 3.5 4. 4.5 5. ]
hs [{'levels': [0.01, 0.03, 0.05, 0.07, 0.1, 0.13, 0.15, 0.2, 0.25, 0.3, 0.35, 0.4, 0.45, 0.5]}, {'title': 'Snow Depth (m)'}]
[0.01 0.03 0.05 0.07 0.1 0.13 0.15 0.2 0.25 0.3 0.35 0.4 0.45 0.5 ]
Tsfc [{'levels': [-40.0, -37.0, -34.0, -31.0, -28.0, -25.0, -22.0, -19.0, -16.0, -13.0, -10.0, -5.0, -3.0, -1.0]}, {'title': 'Surface Temperature (C)'}]
[-40. -37. -34. -31. -28. -25. -22. -19. -16. -13. -10. -5. -3. -1.]
albsni [{'levels': [5, 10, 15, 20, 30, 40, 50, 60, 65, 70, 75, 80, 85, 90]}, {'title': 'Snow Ice Albedo'}]
[ 5 10 15 20 30 40 50 60 65 70 75 80 85 90]
flat [{'levels': [-18.0, -16.0, -14.0, -12.0, -10.0, -8.0, -6.0, -5.0, -4.0, -3.0, -2.0, -1.0, 0.0, 2.0]}, {'title': 'Latent Heat Flux (W/m^2}'}]
[-18. -16. -14. -12. -10. -8. -6. -5. -4. -3. -2. -1. 0. 2.]
fsens [{'levels': [-30.0, -25.0, -20.0, -15.0, -10.0, -5.0, -2.5, 0, 2.5, 5, 10, 15, 20, 25]}, {'title': 'Sensible Heat Flux (W/m^2)'}]
[-30. -25. -20. -15. -10. -5. -2.5 0. 2.5 5. 10. 15.
20. 25. ]
congel [{'levels': [0, 0.5, 1, 1.5, 2, 2.5, 3, 4, 5, 6, 7, 8, 9, 10]}, {'title': 'Congelation growth (cm/day)'}]
[ 0. 0.5 1. 1.5 2. 2.5 3. 4. 5. 6. 7. 8. 9. 10. ]
frazil [{'levels': [0.0, 0.02, 0.04, 0.06, 0.08, 0.1, 0.12, 0.14, 0.16, 0.18, 0.2, 0.22, 0.24, 0.26]}, {'title': 'Frazil growth (cm/day)'}]
[0. 0.02 0.04 0.06 0.08 0.1 0.12 0.14 0.16 0.18 0.2 0.22 0.24 0.26]
snoice [{'levels': [0.0, 0.01, 0.02, 0.03, 0.04, 0.05, 0.06, 0.08, 0.1, 0.12, 0.14, 0.16, 0.18, 0.2]}, {'title': 'Snow-ice growth (cm/day)'}]
[0. 0.01 0.02 0.03 0.04 0.05 0.06 0.08 0.1 0.12 0.14 0.16 0.18 0.2 ]
meltb [{'levels': [0.05, 0.1, 0.25, 0.5, 0.75, 1.0, 1.5, 2.0, 2.5, 3.0, 3.5, 4.0, 4.5, 5.0]}, {'title': 'Bottom Melt (cm/day)'}]
[0.05 0.1 0.25 0.5 0.75 1. 1.5 2. 2.5 3. 3.5 4. 4.5 5. ]
meltt [{'levels': [0.05, 0.1, 0.2, 0.3, 0.4, 0.5, 0.6, 0.7, 0.8, 0.9, 1.0, 1.1, 1.2, 1.3]}, {'title': 'Top Melt (cm/day)'}]
[0.05 0.1 0.2 0.3 0.4 0.5 0.6 0.7 0.8 0.9 1. 1.1 1.2 1.3 ]
meltl [{'levels': [0.01, 0.04, 0.08, 0.12, 0.16, 0.2, 0.24, 0.28, 0.32, 0.36, 0.4, 0.44, 0.48, 0.52]}, {'title': 'Lateral Melt (cm/day)'}]
[0.01 0.04 0.08 0.12 0.16 0.2 0.24 0.28 0.32 0.36 0.4 0.44 0.48 0.52]
dvidtt [{'levels': [-3.6, -3.0, -2.4, -1.8, -1.2, -0.6, 0.0, 0.6, 1.2, 1.8, 2.4, 3.0, 3.6, 4.0]}, {'title': 'Volume tendency due to thermodynamics (cm/day)'}]
[-3.6 -3. -2.4 -1.8 -1.2 -0.6 0. 0.6 1.2 1.8 2.4 3. 3.6 4. ]
dvidtd [{'levels': [-3.6, -3.0, -2.4, -1.8, -1.2, -0.6, 0.0, 0.6, 1.2, 1.8, 2.4, 3.0, 3.6, 4.0]}, {'title': 'Volume tendency due to dynamics (cm/day)'}]
[-3.6 -3. -2.4 -1.8 -1.2 -0.6 0. 0.6 1.2 1.8 2.4 3. 3.6 4. ]
daidtt [{'levels': [-3.6, -3.0, -2.4, -1.8, -1.2, -0.6, 0.0, 0.6, 1.2, 1.8, 2.4, 3.0, 3.6, 4.0]}, {'title': 'Area tendency due to thermodynamics (%/day)'}]
[-3.6 -3. -2.4 -1.8 -1.2 -0.6 0. 0.6 1.2 1.8 2.4 3. 3.6 4. ]
daidtd [{'levels': [-3.6, -3.0, -2.4, -1.8, -1.2, -0.6, 0.0, 0.6, 1.2, 1.8, 2.4, 3.0, 3.6, 4.0]}, {'title': 'Area tendency due to dynamics (%/day)'}]
[-3.6 -3. -2.4 -1.8 -1.2 -0.6 0. 0.6 1.2 1.8 2.4 3. 3.6 4. ]
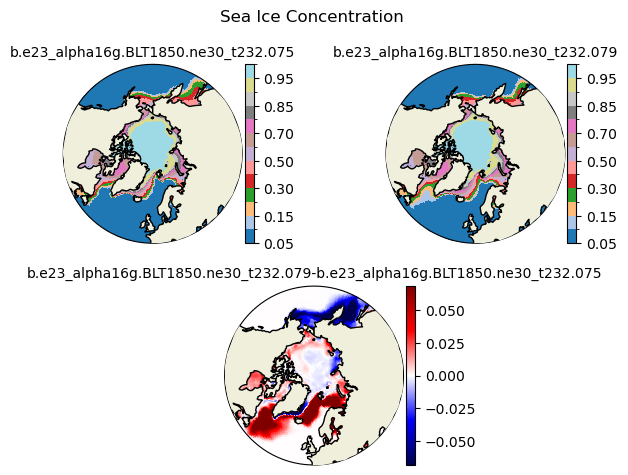
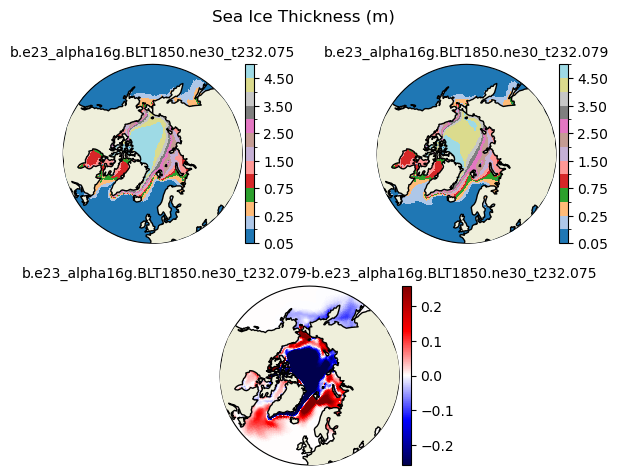
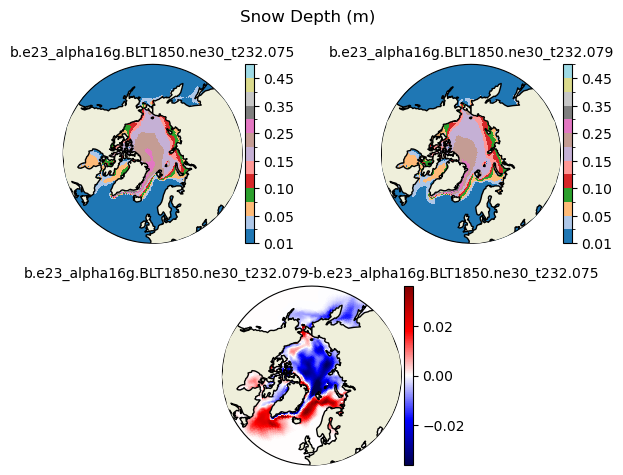
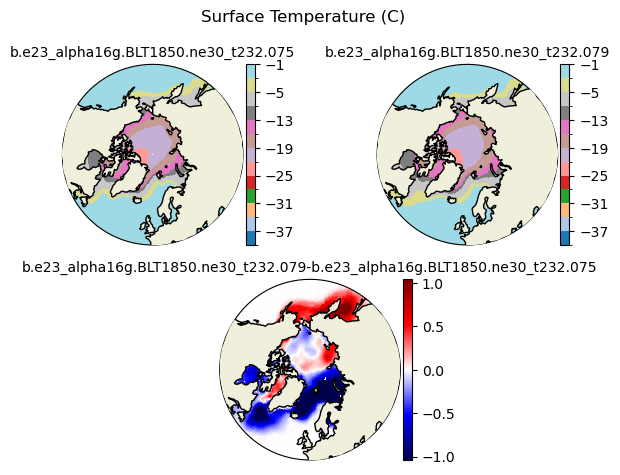
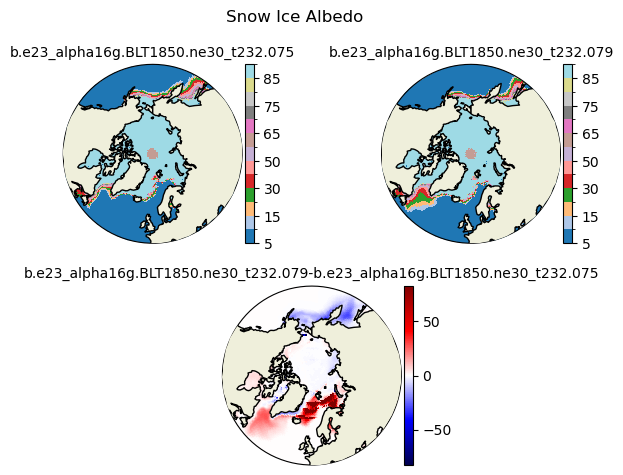
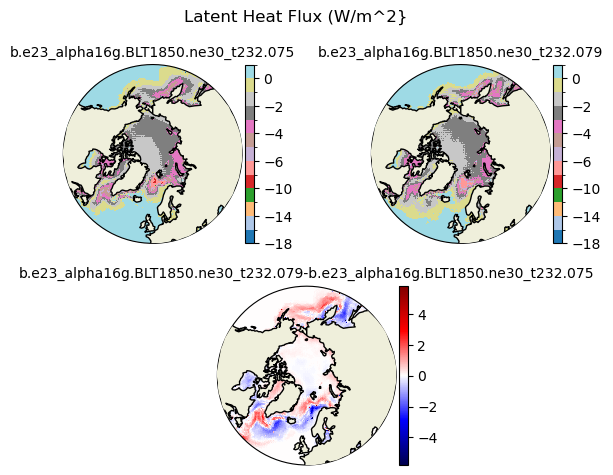
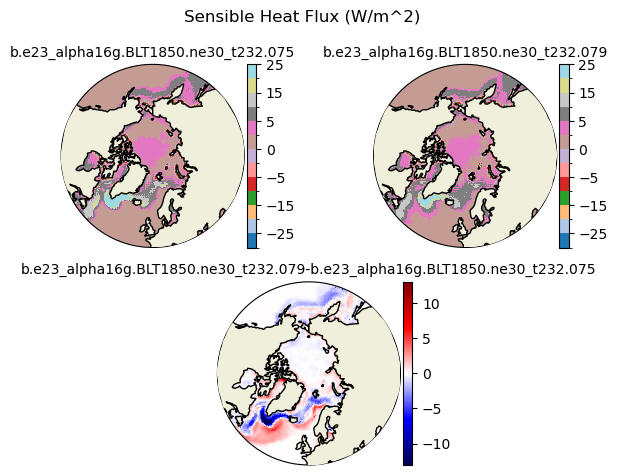
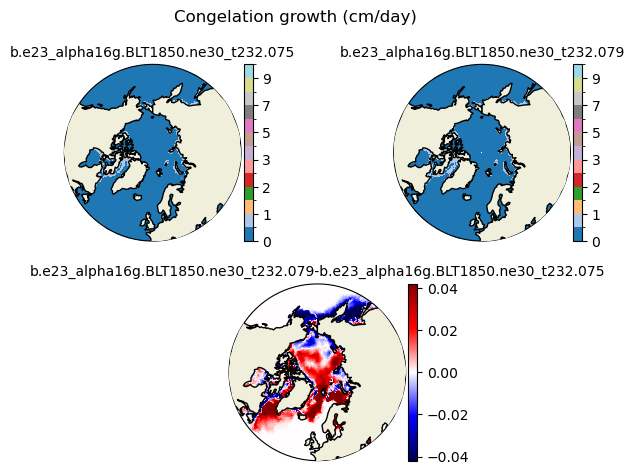
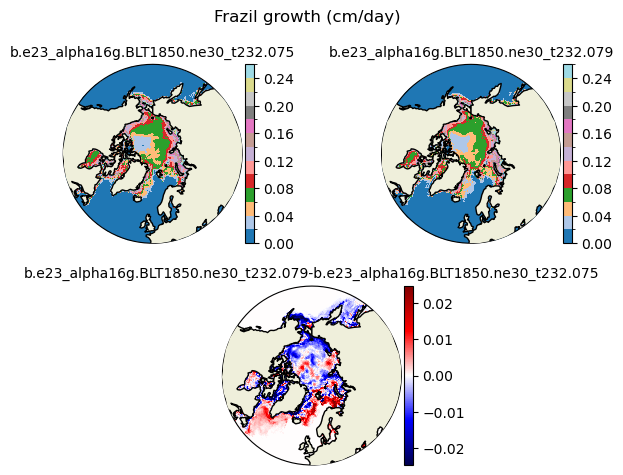
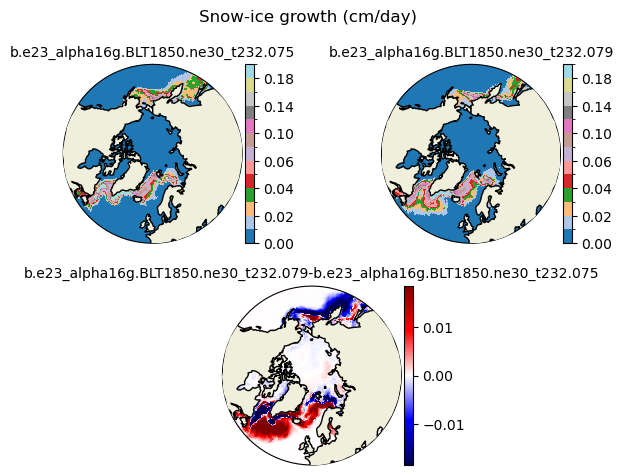
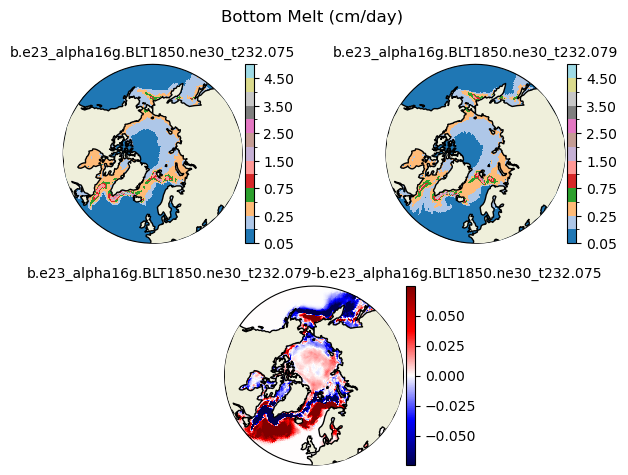
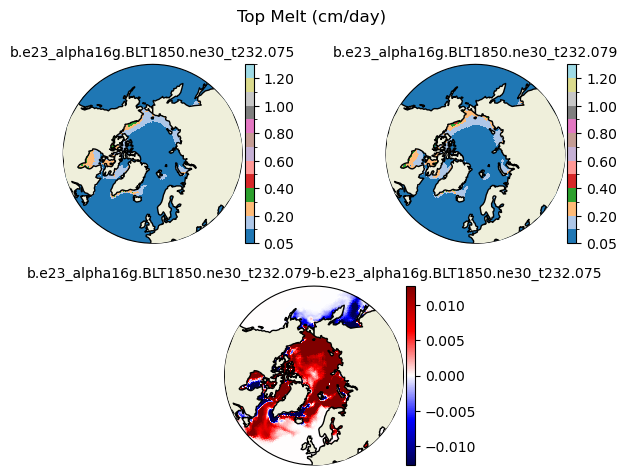
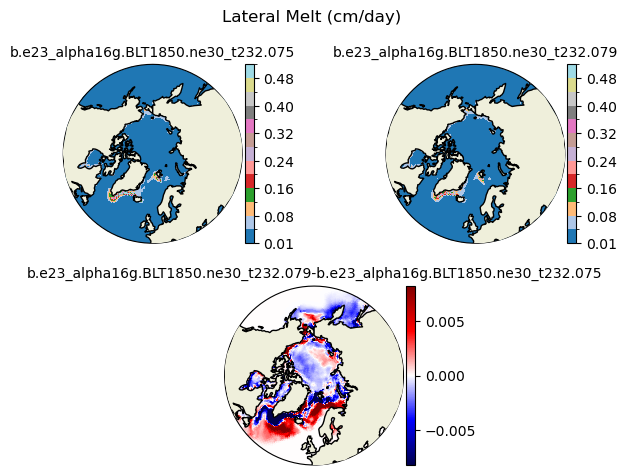
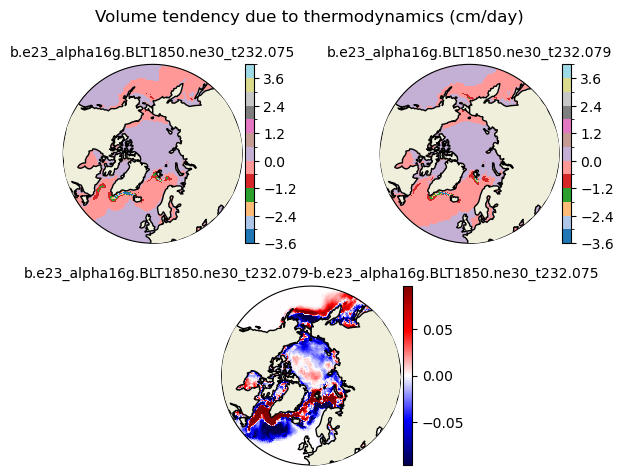
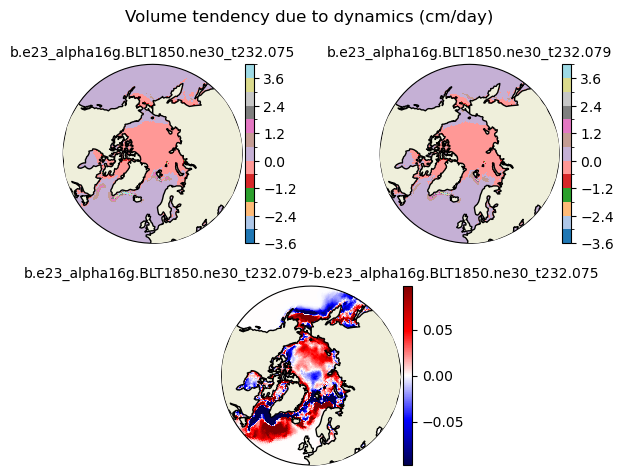
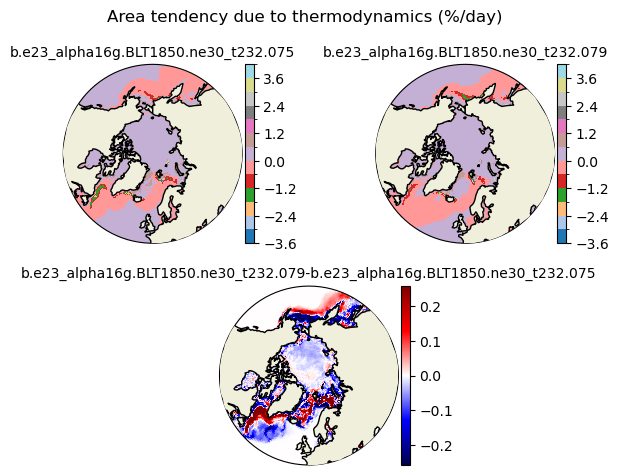
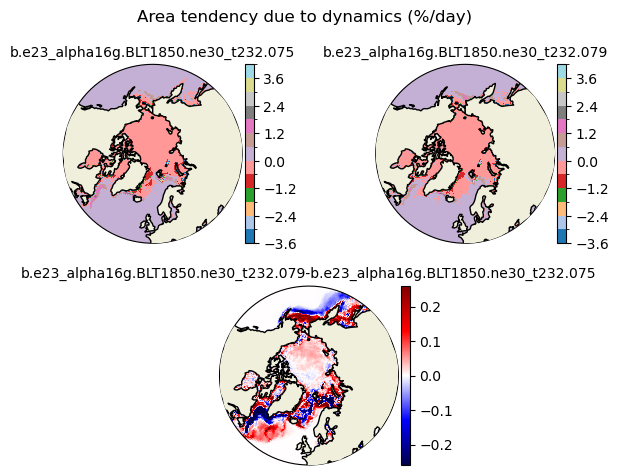
for var in cice_vars:
print(var,cice_vars[var])
vmin=cice_vars[var][0]['levels'][0]
vmax=cice_vars[var][0]['levels'][1]
levels = np.array(cice_vars[var][0]['levels'])
print(levels)
title=cice_vars[var][1]['title']
field1 = ds1_ann[var].isel(time=slice(-nyears,None)).mean("time").squeeze()
field2 = ds2_ann[var].isel(time=slice(-nyears,None)).mean("time").squeeze()
plot_diff(field1,field2,levels,case1,case2,title,"S")
aice [{'levels': [0.05, 0.1, 0.15, 0.2, 0.3, 0.4, 0.5, 0.6, 0.7, 0.8, 0.85, 0.9, 0.95, 0.99]}, {'title': 'Sea Ice Concentration'}]
[0.05 0.1 0.15 0.2 0.3 0.4 0.5 0.6 0.7 0.8 0.85 0.9 0.95 0.99]
hi [{'levels': [0.05, 0.1, 0.25, 0.5, 0.75, 1.0, 1.5, 2.0, 2.5, 3.0, 3.5, 4.0, 4.5, 5.0]}, {'title': 'Sea Ice Thickness (m)'}]
[0.05 0.1 0.25 0.5 0.75 1. 1.5 2. 2.5 3. 3.5 4. 4.5 5. ]
hs [{'levels': [0.01, 0.03, 0.05, 0.07, 0.1, 0.13, 0.15, 0.2, 0.25, 0.3, 0.35, 0.4, 0.45, 0.5]}, {'title': 'Snow Depth (m)'}]
[0.01 0.03 0.05 0.07 0.1 0.13 0.15 0.2 0.25 0.3 0.35 0.4 0.45 0.5 ]
Tsfc [{'levels': [-40.0, -37.0, -34.0, -31.0, -28.0, -25.0, -22.0, -19.0, -16.0, -13.0, -10.0, -5.0, -3.0, -1.0]}, {'title': 'Surface Temperature (C)'}]
[-40. -37. -34. -31. -28. -25. -22. -19. -16. -13. -10. -5. -3. -1.]
albsni [{'levels': [5, 10, 15, 20, 30, 40, 50, 60, 65, 70, 75, 80, 85, 90]}, {'title': 'Snow Ice Albedo'}]
[ 5 10 15 20 30 40 50 60 65 70 75 80 85 90]
flat [{'levels': [-18.0, -16.0, -14.0, -12.0, -10.0, -8.0, -6.0, -5.0, -4.0, -3.0, -2.0, -1.0, 0.0, 2.0]}, {'title': 'Latent Heat Flux (W/m^2}'}]
[-18. -16. -14. -12. -10. -8. -6. -5. -4. -3. -2. -1. 0. 2.]
fsens [{'levels': [-30.0, -25.0, -20.0, -15.0, -10.0, -5.0, -2.5, 0, 2.5, 5, 10, 15, 20, 25]}, {'title': 'Sensible Heat Flux (W/m^2)'}]
[-30. -25. -20. -15. -10. -5. -2.5 0. 2.5 5. 10. 15.
20. 25. ]
congel [{'levels': [0, 0.5, 1, 1.5, 2, 2.5, 3, 4, 5, 6, 7, 8, 9, 10]}, {'title': 'Congelation growth (cm/day)'}]
[ 0. 0.5 1. 1.5 2. 2.5 3. 4. 5. 6. 7. 8. 9. 10. ]
frazil [{'levels': [0.0, 0.02, 0.04, 0.06, 0.08, 0.1, 0.12, 0.14, 0.16, 0.18, 0.2, 0.22, 0.24, 0.26]}, {'title': 'Frazil growth (cm/day)'}]
[0. 0.02 0.04 0.06 0.08 0.1 0.12 0.14 0.16 0.18 0.2 0.22 0.24 0.26]
snoice [{'levels': [0.0, 0.01, 0.02, 0.03, 0.04, 0.05, 0.06, 0.08, 0.1, 0.12, 0.14, 0.16, 0.18, 0.2]}, {'title': 'Snow-ice growth (cm/day)'}]
[0. 0.01 0.02 0.03 0.04 0.05 0.06 0.08 0.1 0.12 0.14 0.16 0.18 0.2 ]
meltb [{'levels': [0.05, 0.1, 0.25, 0.5, 0.75, 1.0, 1.5, 2.0, 2.5, 3.0, 3.5, 4.0, 4.5, 5.0]}, {'title': 'Bottom Melt (cm/day)'}]
[0.05 0.1 0.25 0.5 0.75 1. 1.5 2. 2.5 3. 3.5 4. 4.5 5. ]
meltt [{'levels': [0.05, 0.1, 0.2, 0.3, 0.4, 0.5, 0.6, 0.7, 0.8, 0.9, 1.0, 1.1, 1.2, 1.3]}, {'title': 'Top Melt (cm/day)'}]
[0.05 0.1 0.2 0.3 0.4 0.5 0.6 0.7 0.8 0.9 1. 1.1 1.2 1.3 ]
meltl [{'levels': [0.01, 0.04, 0.08, 0.12, 0.16, 0.2, 0.24, 0.28, 0.32, 0.36, 0.4, 0.44, 0.48, 0.52]}, {'title': 'Lateral Melt (cm/day)'}]
[0.01 0.04 0.08 0.12 0.16 0.2 0.24 0.28 0.32 0.36 0.4 0.44 0.48 0.52]
dvidtt [{'levels': [-3.6, -3.0, -2.4, -1.8, -1.2, -0.6, 0.0, 0.6, 1.2, 1.8, 2.4, 3.0, 3.6, 4.0]}, {'title': 'Volume tendency due to thermodynamics (cm/day)'}]
[-3.6 -3. -2.4 -1.8 -1.2 -0.6 0. 0.6 1.2 1.8 2.4 3. 3.6 4. ]
dvidtd [{'levels': [-3.6, -3.0, -2.4, -1.8, -1.2, -0.6, 0.0, 0.6, 1.2, 1.8, 2.4, 3.0, 3.6, 4.0]}, {'title': 'Volume tendency due to dynamics (cm/day)'}]
[-3.6 -3. -2.4 -1.8 -1.2 -0.6 0. 0.6 1.2 1.8 2.4 3. 3.6 4. ]
daidtt [{'levels': [-3.6, -3.0, -2.4, -1.8, -1.2, -0.6, 0.0, 0.6, 1.2, 1.8, 2.4, 3.0, 3.6, 4.0]}, {'title': 'Area tendency due to thermodynamics (%/day)'}]
[-3.6 -3. -2.4 -1.8 -1.2 -0.6 0. 0.6 1.2 1.8 2.4 3. 3.6 4. ]
daidtd [{'levels': [-3.6, -3.0, -2.4, -1.8, -1.2, -0.6, 0.0, 0.6, 1.2, 1.8, 2.4, 3.0, 3.6, 4.0]}, {'title': 'Area tendency due to dynamics (%/day)'}]
[-3.6 -3. -2.4 -1.8 -1.2 -0.6 0. 0.6 1.2 1.8 2.4 3. 3.6 4. ]
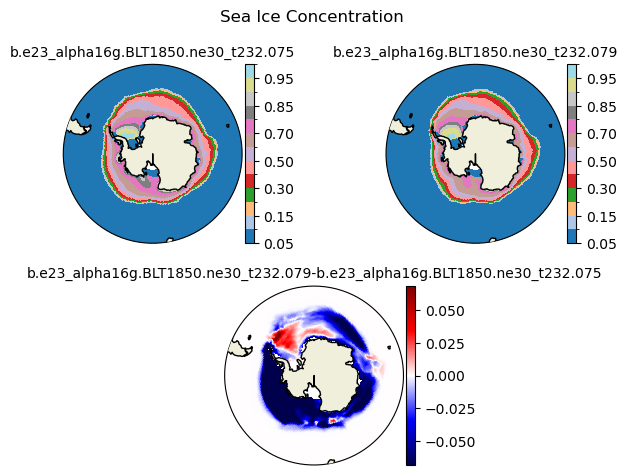
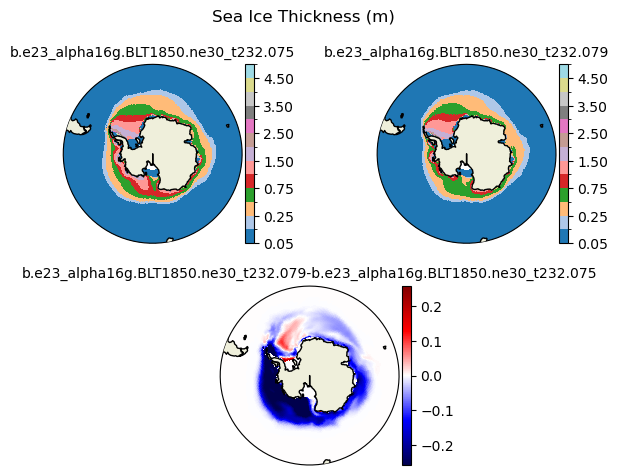
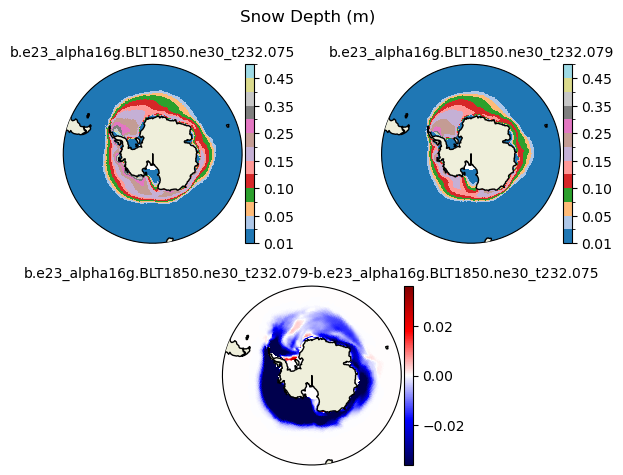
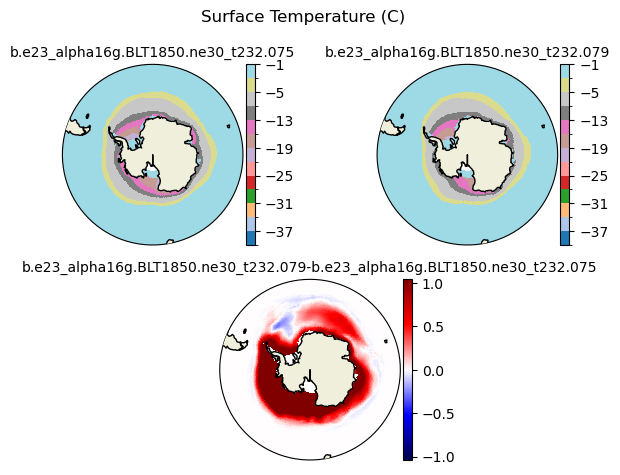
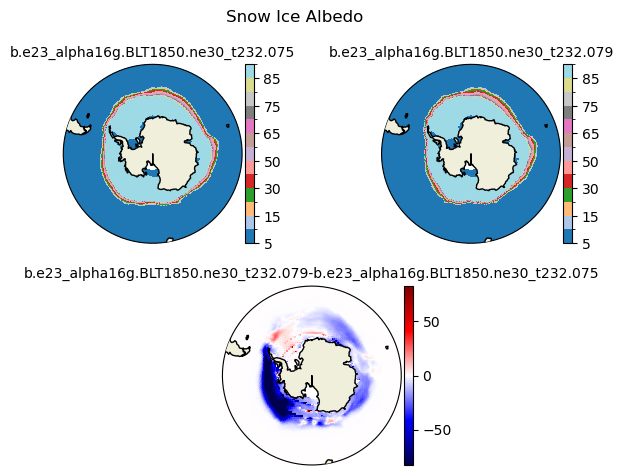
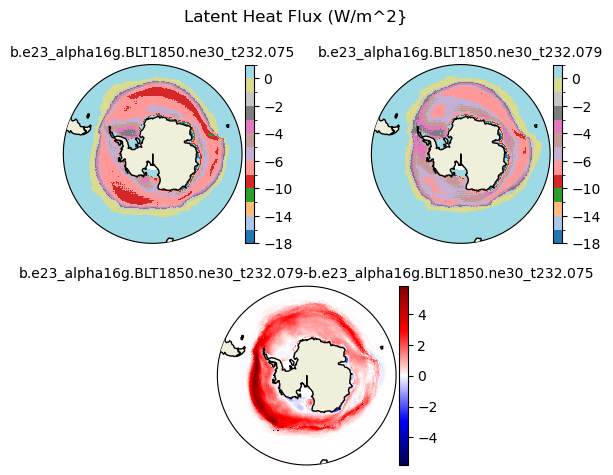
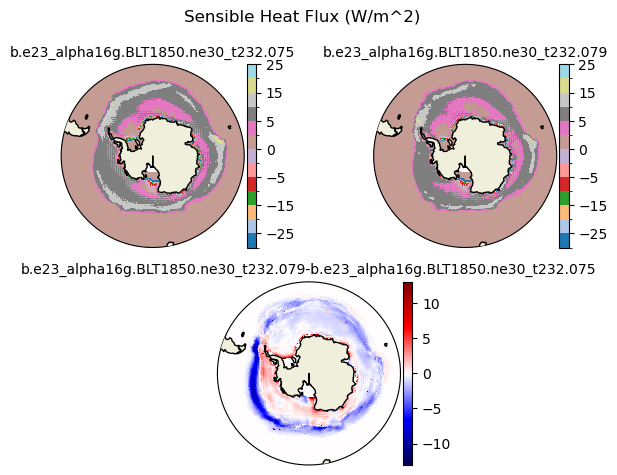
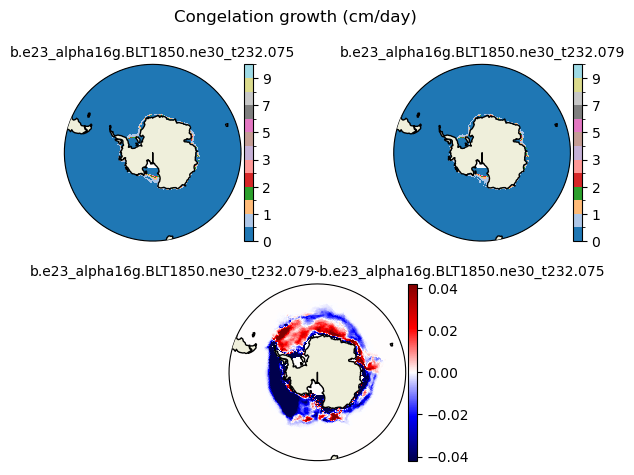
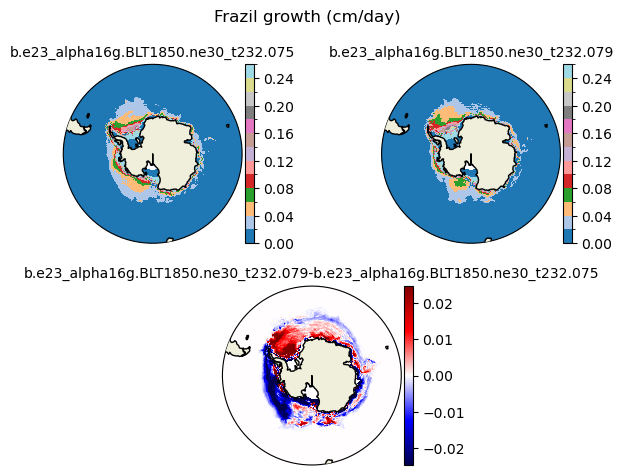
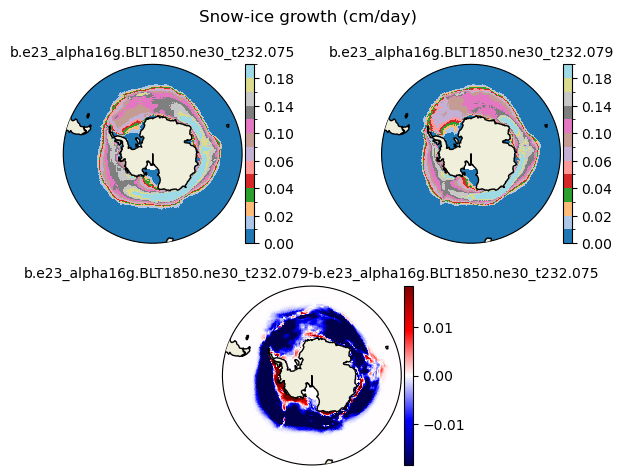
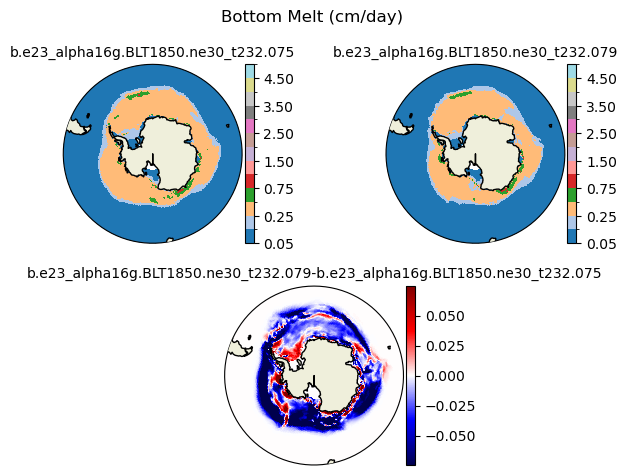
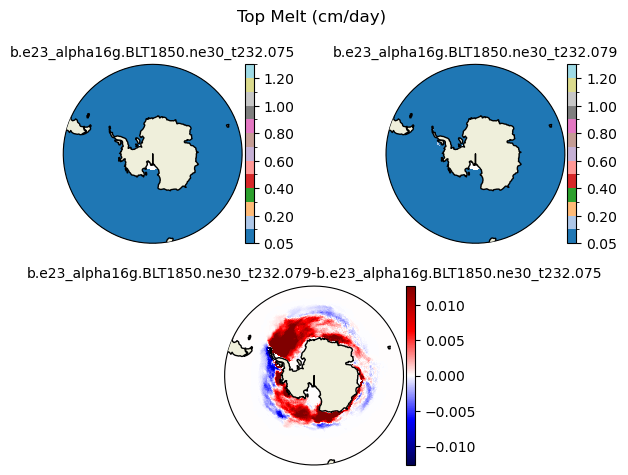
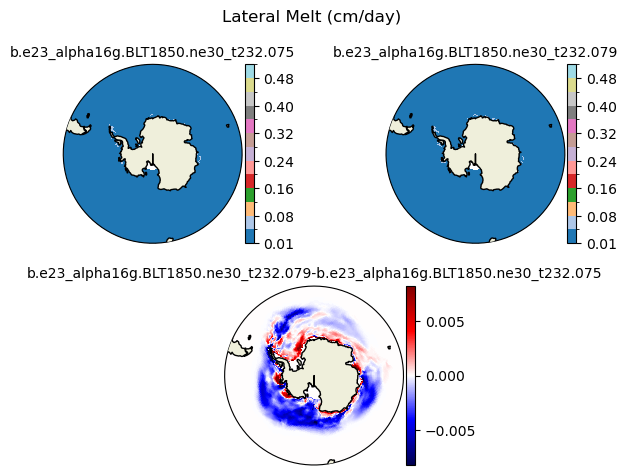
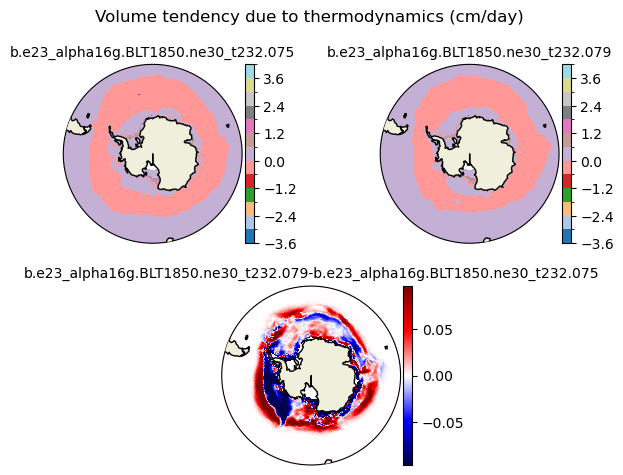
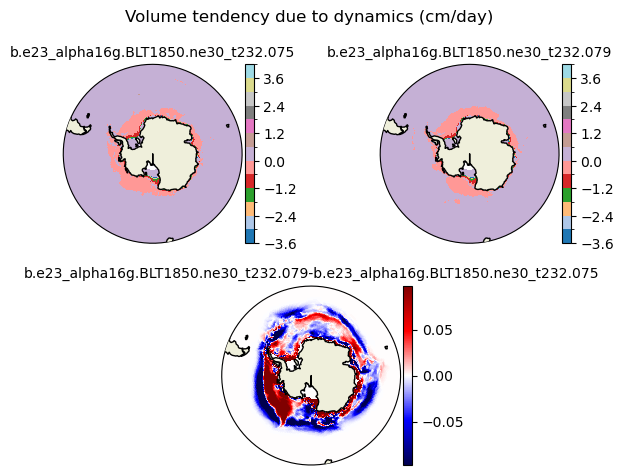
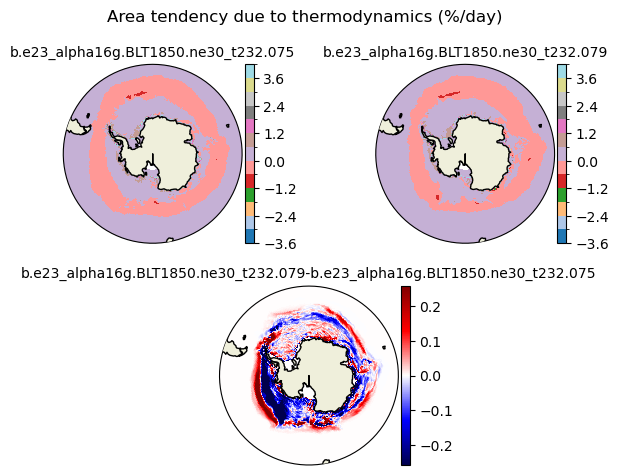
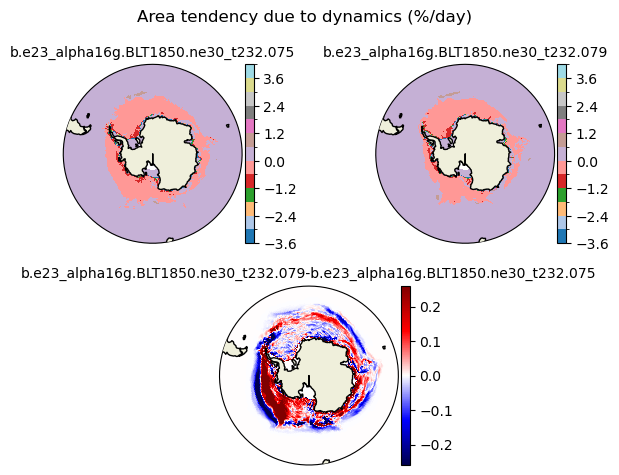
ds1_area = (tarea*ds1.aice).where(TLAT>0).sum(dim=['nj','ni'])*1.0e-12
ds2_area = (tarea*ds2.aice).where(TLAT>0).sum(dim=['nj','ni'])*1.0e-12
ds1_vhi = (tarea*ds1.hi).where(TLAT>0).sum(dim=['nj','ni'])*1.0e-13
ds2_vhi = (tarea*ds2.hi).where(TLAT>0).sum(dim=['nj','ni'])*1.0e-13
ds1_vhs = (tarea*ds1.hs).where(TLAT>0).sum(dim=['nj','ni'])*1.0e-13
ds2_vhs = (tarea*ds2.hs).where(TLAT>0).sum(dim=['nj','ni'])*1.0e-13
fig = plt.figure(figsize=(10,10),tight_layout=True)
ax = fig.add_subplot(3,1,1)
ds1_vhi.plot()
ds2_vhi.plot()
plt.ylim((0,10))
plt.xlabel("Month")
plt.ylabel("NH Sea Ice Volume $m x 10^{13}$")
plt.legend([case1,case2])
ax = fig.add_subplot(3,1,2)
ds1_vhs.plot()
ds2_vhs.plot()
plt.ylim((0,1))
plt.xlabel("Month")
plt.ylabel("NH Snow Volume $m x 10^{13}$")
plt.legend([case1,case2])
ax = fig.add_subplot(3,1,3)
ds1_area.plot()
ds2_area.plot()
plt.ylim((0,25))
plt.xlabel("Month")
plt.ylabel("NH Sea Ice Area $m x 10^{12}$")
plt.legend([case1,case2])
<matplotlib.legend.Legend at 0x7fbad6bf8190>
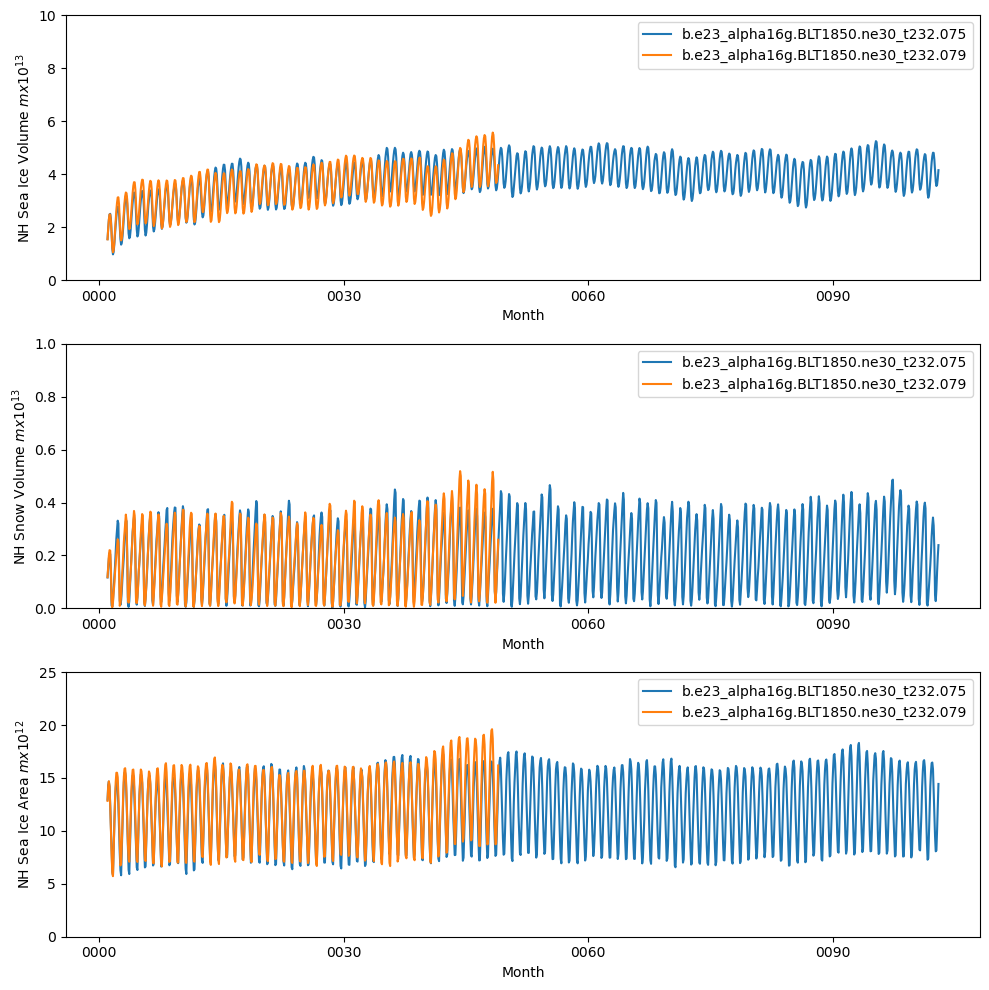
ds1_area_ann = (tarea*ds1_ann['aice']).where(TLAT>0).sum(dim=['nj','ni'])*1.0e-12
ds2_area_ann = (tarea*ds2_ann['aice']).where(TLAT>0).sum(dim=['nj','ni'])*1.0e-12
ds1_vhi_ann = (tarea*ds1_ann['hi']).where(TLAT>0).sum(dim=['nj','ni'])*1.0e-13
ds2_vhi_ann = (tarea*ds2_ann['hi']).where(TLAT>0).sum(dim=['nj','ni'])*1.0e-13
ds1_vhs_ann = (tarea*ds1_ann['hs']).where(TLAT>0).sum(dim=['nj','ni'])*1.0e-13
ds2_vhs_ann = (tarea*ds2_ann['hs']).where(TLAT>0).sum(dim=['nj','ni'])*1.0e-13
fig = plt.figure(figsize=(10,10),tight_layout=True)
ax = fig.add_subplot(3,1,1)
ds1_vhi_ann.plot()
ds2_vhi_ann.plot()
plt.ylim((0,10))
plt.xlabel("Year")
plt.ylabel("NH Annual Mean Sea Ice Volume $m x 10^{13}$")
plt.legend([case1,case2])
ax = fig.add_subplot(3,1,2)
ds1_vhs_ann.plot()
ds2_vhs_ann.plot()
plt.ylim((0,1))
plt.xlabel("Year")
plt.ylabel("NH Annual Mean Snow Volume $m x 10^{13}$")
plt.legend([case1,case2])
ax = fig.add_subplot(3,1,3)
ds1_area_ann.plot()
ds2_area_ann.plot()
plt.ylim((0,25))
plt.xlabel("Year")
plt.ylabel("NH Annual Mean Sea Ice Area $m x 10^{12}$")
plt.legend([case1,case2])
<matplotlib.legend.Legend at 0x7fbadb201610>
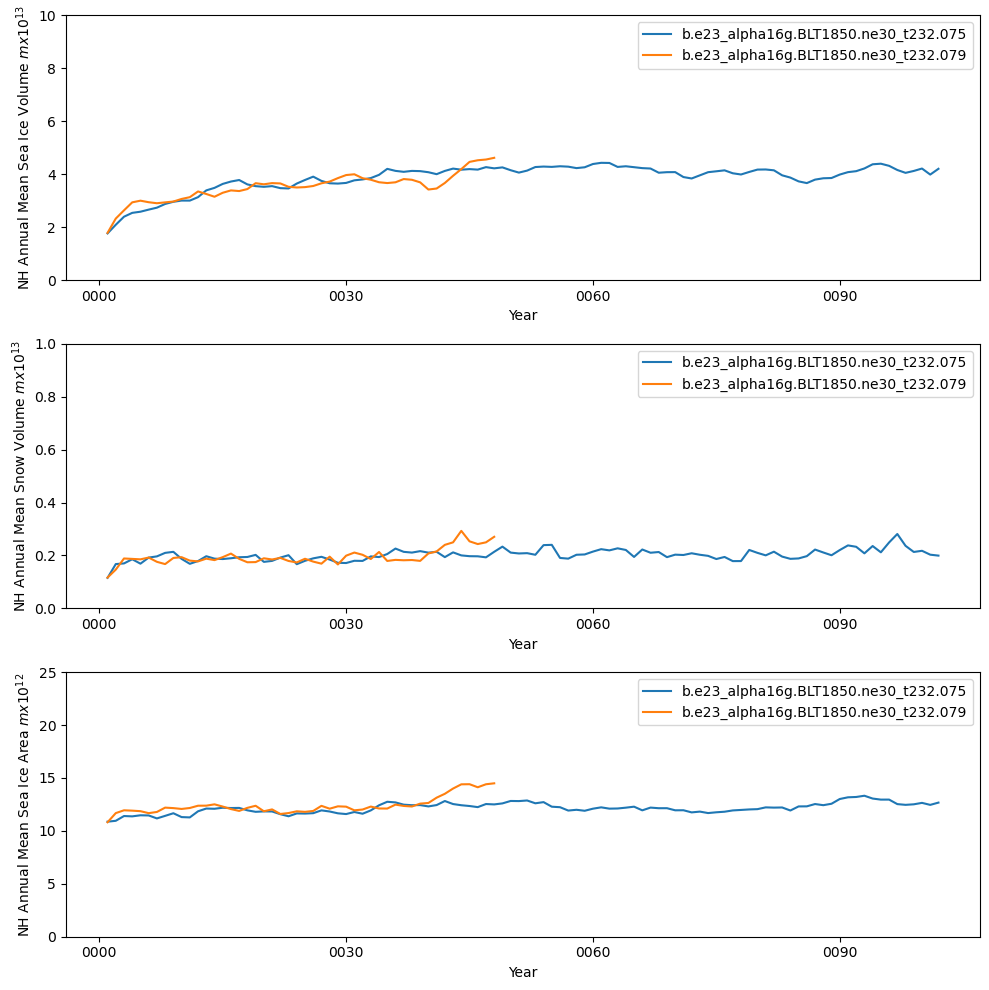
ds1_area.sel(time=ds1_area.time.dt.month.isin([10])).plot() ds2_area.sel(time=ds2_area.time.dt.month.isin([10])).plot()
plt.ylim((0,25)) plt.xlabel(“Year”) plt.ylabel(“NH September Sea Ice Area \(m x 10^{12}\)”) plt.legend([case1,case2])
ds1_area = (tarea*ds1.aice).where(TLAT<0).sum(dim=['nj','ni'])*1.0e-12
ds2_area = (tarea*ds2.aice).where(TLAT<0).sum(dim=['nj','ni'])*1.0e-12
ds1_area.plot()
ds2_area.plot()
plt.ylim((0,25))
plt.xlabel("Month")
plt.ylabel("SH Sea Ice Area $m x 10^{12}$")
plt.legend([case1,case2])
<matplotlib.legend.Legend at 0x7fbaf9399610>
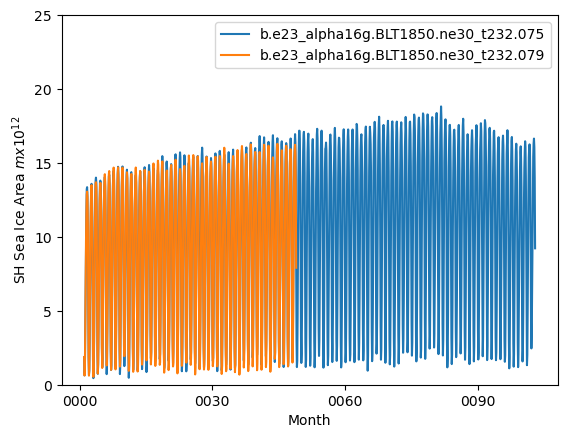
ds1_area_ann = (tarea*ds1_ann.aice).where(TLAT<0).sum(dim=['nj','ni'])*1.0e-12
ds2_area_ann = (tarea*ds2_ann.aice).where(TLAT<0).sum(dim=['nj','ni'])*1.0e-12
ds1_area_ann.plot()
ds2_area_ann.plot()
plt.ylim((0,25))
plt.xlabel("Year")
plt.ylabel("SH Annual Mean Sea Ice Area $m x 10^{12}$")
plt.legend([case1,case2])
<matplotlib.legend.Legend at 0x7fbadc1b3f50>
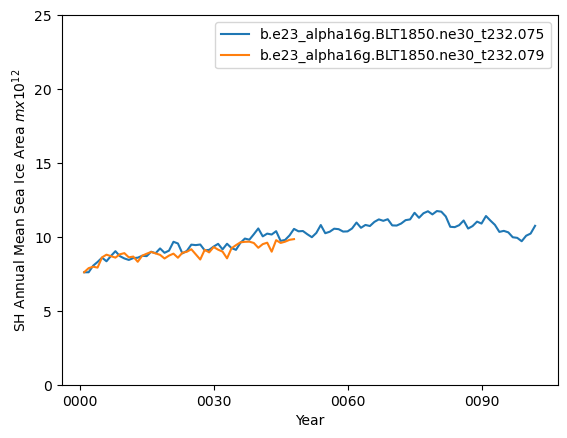
### Read in the NSIDC data from files
path_nsidc = '/glade/campaign/cesm/development/pcwg/ice/data/NSIDC_SeaIce_extent/'
jan_nsidc = pd.read_csv(path_nsidc+'N_01_extent_v3.0.csv',na_values=['-99.9'])
feb_nsidc = pd.read_csv(path_nsidc+'N_02_extent_v3.0.csv',na_values=['-99.9'])
mar_nsidc = pd.read_csv(path_nsidc+'N_03_extent_v3.0.csv',na_values=['-99.9'])
apr_nsidc = pd.read_csv(path_nsidc+'N_04_extent_v3.0.csv',na_values=['-99.9'])
may_nsidc = pd.read_csv(path_nsidc+'N_05_extent_v3.0.csv',na_values=['-99.9'])
jun_nsidc = pd.read_csv(path_nsidc+'N_06_extent_v3.0.csv',na_values=['-99.9'])
jul_nsidc = pd.read_csv(path_nsidc+'N_07_extent_v3.0.csv',na_values=['-99.9'])
aug_nsidc = pd.read_csv(path_nsidc+'N_08_extent_v3.0.csv',na_values=['-99.9'])
sep_nsidc = pd.read_csv(path_nsidc+'N_09_extent_v3.0.csv',na_values=['-99.9'])
oct_nsidc = pd.read_csv(path_nsidc+'N_10_extent_v3.0.csv',na_values=['-99.9'])
nov_nsidc = pd.read_csv(path_nsidc+'N_11_extent_v3.0.csv',na_values=['-99.9'])
dec_nsidc = pd.read_csv(path_nsidc+'N_12_extent_v3.0.csv',na_values=['-99.9'])
jan_area = jan_nsidc.iloc[:,5].values
feb_area = feb_nsidc.iloc[:,5].values
mar_area = mar_nsidc.iloc[:,5].values
apr_area = apr_nsidc.iloc[:,5].values
may_area = may_nsidc.iloc[:,5].values
jun_area = jun_nsidc.iloc[:,5].values
jul_area = jul_nsidc.iloc[:,5].values
aug_area = aug_nsidc.iloc[:,5].values
sep_area = sep_nsidc.iloc[:,5].values
oct_area = oct_nsidc.iloc[:,5].values
nov_area = nov_nsidc.iloc[:,5].values
dec_area = dec_nsidc.iloc[:,5].values
jan_ext = jan_nsidc.iloc[:,4].values
feb_ext = feb_nsidc.iloc[:,4].values
mar_ext = mar_nsidc.iloc[:,4].values
apr_ext = apr_nsidc.iloc[:,4].values
may_ext = may_nsidc.iloc[:,4].values
jun_ext = jun_nsidc.iloc[:,4].values
jul_ext = jul_nsidc.iloc[:,4].values
aug_ext = aug_nsidc.iloc[:,4].values
sep_ext = sep_nsidc.iloc[:,4].values
oct_ext = oct_nsidc.iloc[:,4].values
nov_ext = nov_nsidc.iloc[:,4].values
dec_ext = dec_nsidc.iloc[:,4].values
print(dec_ext)
nsidc_clim = [np.nanmean(jan_ext[0:35]),np.nanmean(feb_ext[0:35]),np.nanmean(mar_ext[0:35]),np.nanmean(apr_ext[0:35]),
np.nanmean(may_ext[0:35]),np.nanmean(jun_ext[0:35]),np.nanmean(jul_ext[0:35]),np.nanmean(aug_ext[0:35]),
np.nanmean(sep_ext[0:35]),np.nanmean(oct_ext[0:35]),np.nanmean(nov_ext[0:35]),np.nanmean(dec_ext[0:35])]
plt.plot(nsidc_clim)
[13.67 13.34 13.59 13.34 13.64 13.3 12.99 13.05 13.22 nan 13.63 13.39
13.11 12.95 13.41 13.32 13.27 12.92 12.86 13.08 12.76 12.64 12.64 12.49
12.61 12.59 12.55 12.23 11.95 12.03 12.36 12.2 11.83 12.15 12.01 12.18
12.35 12.04 11.46 11.74 11.86]
[<matplotlib.lines.Line2D at 0x7fbade150350>]
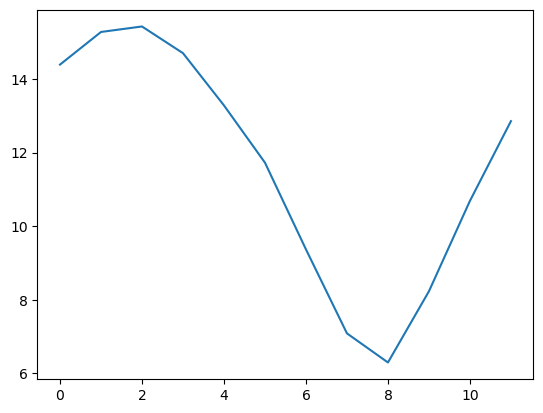
aice1_month = ds1['aice'].groupby("time.month").mean(dim="time",skipna=True)
aice2_month = ds2['aice'].groupby("time.month").mean(dim="time",skipna=True)
mask_tmp1 = np.where(np.logical_and(aice1_month > 0.15, ds1['TLAT'] > 0), 1., 0.)
mask_tmp2 = np.where(np.logical_and(aice2_month > 0.15, ds1['TLAT'] > 0), 1., 0.)
mask_ext1 = xr.DataArray(data=mask_tmp1,dims=["month","nj", "ni"])
mask_ext2 = xr.DataArray(data=mask_tmp2,dims=["month","nj", "ni"])
ext1 = (mask_ext1*tarea).sum(['ni','nj'])*1.0e-12
ext2 = (mask_ext2*tarea).sum(['ni','nj'])*1.0e-12
plt.plot(ext1)
plt.plot(ext2)
plt.plot(nsidc_clim)
plt.ylim((0,25))
plt.xlabel("Month")
plt.ylabel("Climatological Seasonal Cycle Ice Extent $m x 10^{12}$")
plt.legend([case1,case2,"NSIDC"])
/glade/work/dbailey/conda-envs/cupid-analysis/lib/python3.11/site-packages/distributed/client.py:3162: UserWarning: Sending large graph of size 24.99 MiB.
This may cause some slowdown.
Consider scattering data ahead of time and using futures.
warnings.warn(
<matplotlib.legend.Legend at 0x7fbade302e10>
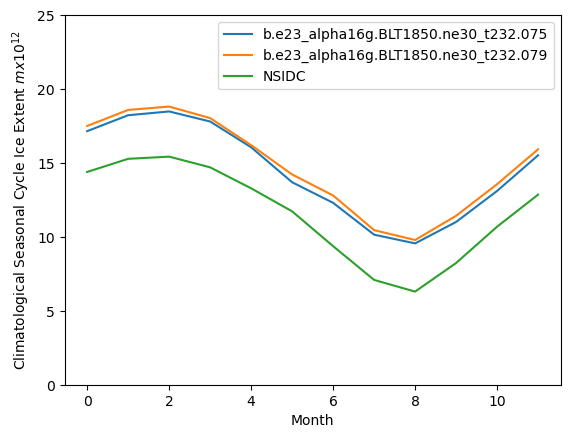
ds1_area = (tarea*ds1.aice).where(TLAT>0).sum(dim=['nj','ni'])*1.0e-12
ds2_area = (tarea*ds2.aice).where(TLAT>0).sum(dim=['nj','ni'])*1.0e-12
ds1_sep = ds1_area.sel(time=(ds1_area.time.dt.month == 9))
ds2_sep = ds2_area.sel(time=(ds2_area.time.dt.month == 9))
plt.plot(ds1_sep)
plt.plot(ds2_sep)
plt.plot(sep_area)
plt.ylim((0,25))
plt.xlabel("Year")
plt.ylabel("Sea Ice Area $mx10^{12}$")
plt.legend([case1,case2,"NSIDC"])
<matplotlib.legend.Legend at 0x7fbabfaf3490>
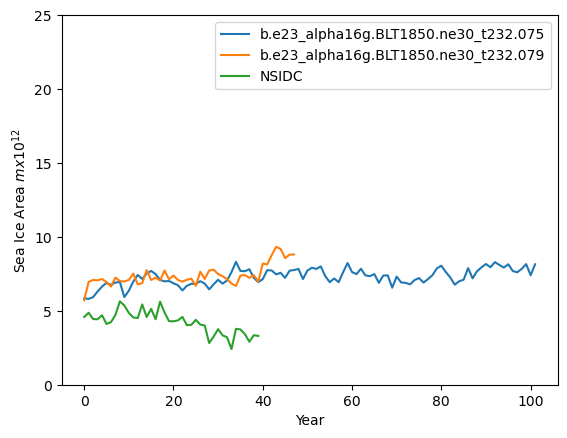
latm = cice_masks['Lab_lat']
lonm = cice_masks['Lab_lon']
lon = np.where(TLON < 0, TLON+360.,TLON)
mask1 = np.where(np.logical_and(TLAT > latm[0], TLAT < latm[1]),1.,0.)
mask2 = np.where(np.logical_or(lon > lonm[0], lon < lonm[1]),1.,0.)
mask = mask1*mask2
ds1_lab = (mask*tarea*ds1.aice).sum(dim=['nj','ni'])*1.0e-12
ds2_lab = (mask*tarea*ds2.aice).sum(dim=['nj','ni'])*1.0e-12
ds1_lab.plot()
ds2_lab.plot()
plt.ylim((0,10))
plt.xlabel("Month")
plt.ylabel("Labrador Sea Ice Area $m x 10^{12}$")
plt.legend([case1,case2])
<matplotlib.legend.Legend at 0x7fbade16ba90>
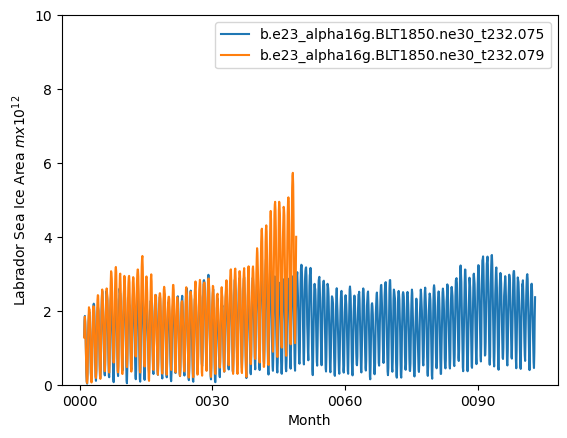
uvel1 = ds1_ann['uvel'].isel(time=slice(-nyears,None)).mean("time").squeeze()
vvel1 = ds1_ann['vvel'].isel(time=slice(-nyears,None)).mean("time").squeeze()
uvel2 = ds2_ann['uvel'].isel(time=slice(-nyears,None)).mean("time").squeeze()
vvel2 = ds2_ann['vvel'].isel(time=slice(-nyears,None)).mean("time").squeeze()
ds_angle = xr.open_dataset("/glade/derecho/scratch/dbailey/ADF/b.e23_alpha16g.BLT1850.ne30_t232.075c/ts/angle.nc")
angle = ds_angle['ANGLE']
vect_diff(uvel1,vvel1,uvel2,vvel2,angle,"N")
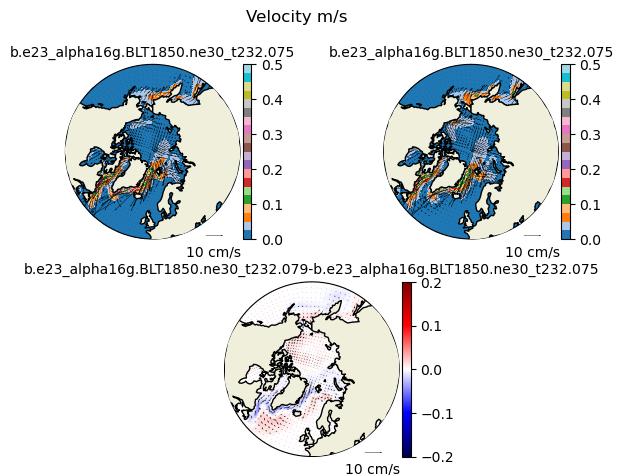
vect_diff(uvel1,vvel1,uvel2,vvel2,angle,"S")
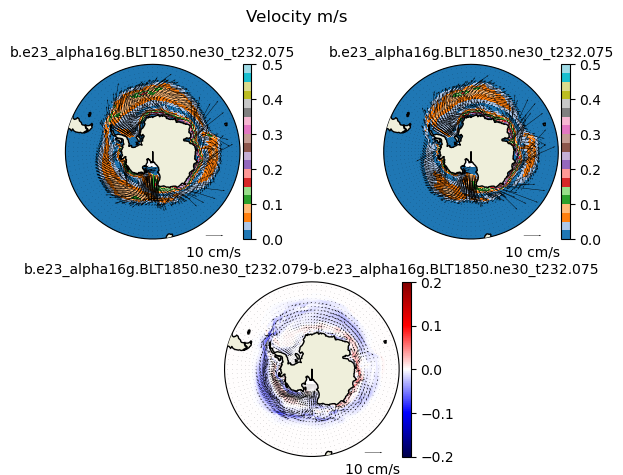